Mapping the Mountains and Valleys of the Mind
Can an understanding of the neural landscape help us navigate the flow of our thoughts?
This article appeared in the second volume of the zine MIND OCEAN SPACE which you should definitely check out! The article is on page 32 - but the whole zine is awesome and is packed with beautiful writing, poetry, art and music. A full pdf is available for free through the provided link.
Imagine that you’re waiting for a text back from someone, and that this situation is causing you some anxiety (this may not be very hard to imagine). Your awareness becomes flooded with a series of thoughts related to this predicament. “Why haven’t they texted back yet? What could possibly be preventing them from responding? Am I not important enough to be responded to? Are they mad at me?” Without interruption, these kinds of thoughts can persist for quite some time. Then, a little while later, you snap out of it—you’ve been caught in an unintended thought loop.
This kind of fixated negative thinking is extremely common, and a hallmark of mood disorders1–3. Given the importance of understanding this kind of thinking, a natural place to look for insight is the brain. What’s happening in your brain when you’re thinking about something? How does the brain give rise to different patterns of thinking, like the fixed thinking illustrated above? To dive into this question, we need to find a way to represent what’s happening in the brain in an intuitive way. The starting point for this is what’s known as _neural activity_. Neural activity is typically any electrical activity happening in the brain, which we can measure in a few different ways. For instance, functional magnetic resonance imaging (fMRI) measures blood flow to different regions of the brain, because more blood is needed where neurons are firing more. Electroencephalography, or EEG, instead directly measures electrical activity generated by different parts of the brain. EEG involves placing electrodes at varying locations across the scalp.
Often—and especially when we’re thinking about aspects of cognition that are temporal in nature, such as the flow of thoughts—we’re interested in how neural activity changes over time (this is also called neural dynamics). To illustrate how neural dynamics are conceptualised, let’s consider a motivating example. Say we’re recording EEG from three electrodes across our participant’s scalp, and that we are measuring the electrical activity at each electrode every millisecond (or at a 1 kHz sampling rate, for all you frequency fiends). While one could analyse each electrode individually, let’s instead think about not just each electrode’s individual changes, but how all the electrodes are changing in relation to each other. To do this, consider a three-dimensional space where each axis is the electrical activity recorded at one of our three electrodes.
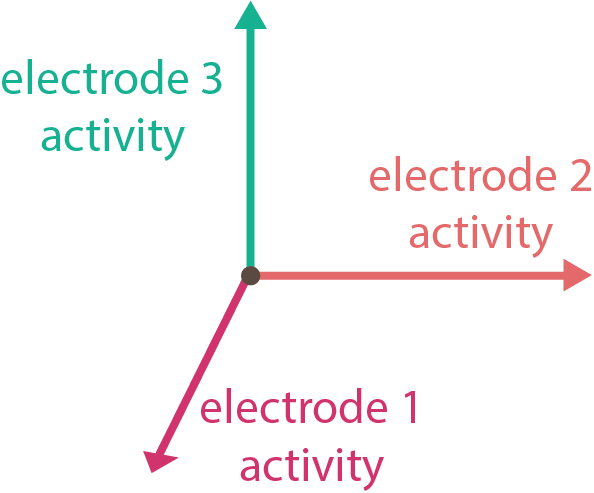
Figure 1. A three dimensional space where each axis is the activity of one electrode. This space enables us to visualise how electrode activities vary together, rahter than considering each electrode separately.
ASIDE: In practice of course, we have many more electrodes than just three, often on the order of one hundred. In these cases, researchers use mathematically-guided approaches to visualise what’s happening in these hundred-dimensional spaces as if they were three dimensional4. Contrary to popular belief, not even mathematicians can visualise things in more than three dimensions. This is merely a technical note, though, for completeness—for our purposes, you can go ahead and think of the state space as simply consisting of three dimensions, each corresponding to the activity of one electrode.
This 3-dimensional space can also be called a state space, or sometimes, a neural state space. Now, at each millisecond, we can plot the activity we recorded at each of our three electrodes as a point in this space, where the distance along each axis is determined by the activity of the corresponding electrode.
Trajectories and points in neural state space often exist on a “landscape,” or a structured object, such as a line, plane, or a ring5. Head direction cells (ie. neurons indicating to the brain which direction the head is facing) form a ring in neural state space. Each place along the ring encodes a particular direction the animal’s head could be facing.
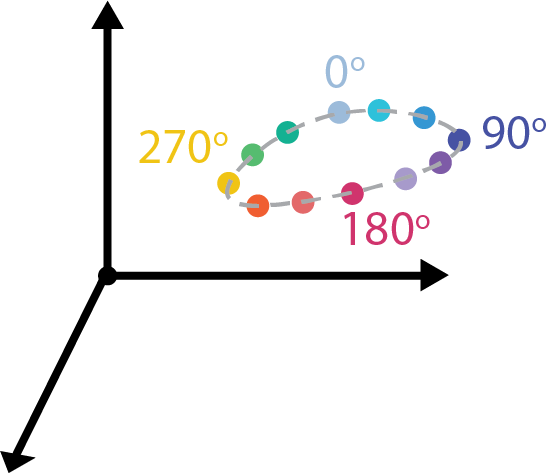
Figure 2. Head direction cells form a ring in state space. The position along the ring corresponds to the angle the head is facing.
Now, if we go back to our electrodes measuring electrical activity over time, we can trace out a path, or a trajectory, through the state space.
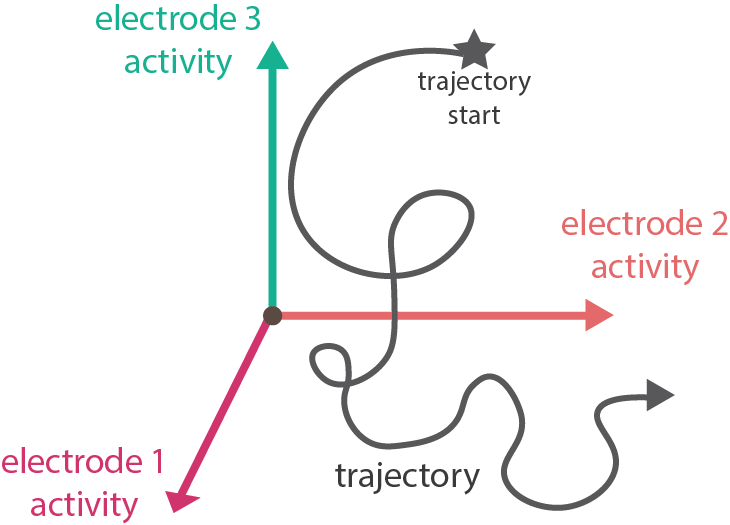
Figure 3. A trajectory in state space: at each time point in the recording, we plot a point corresponding to each of the electrodes’ activity at that time point. Over the course of the recording, this traces out a trajectory in state space.
We can look for patterns in these trajectories, and ask how this relates to the behaviour being performed. For instance, when monkeys reach for objects, their corresponding neural trajectories rotate in neural state space6. Different rotations correspond to different reaches.
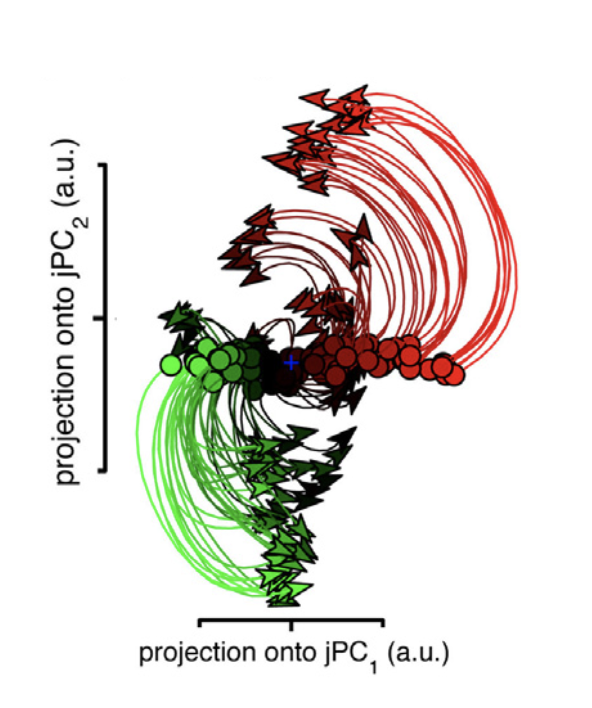
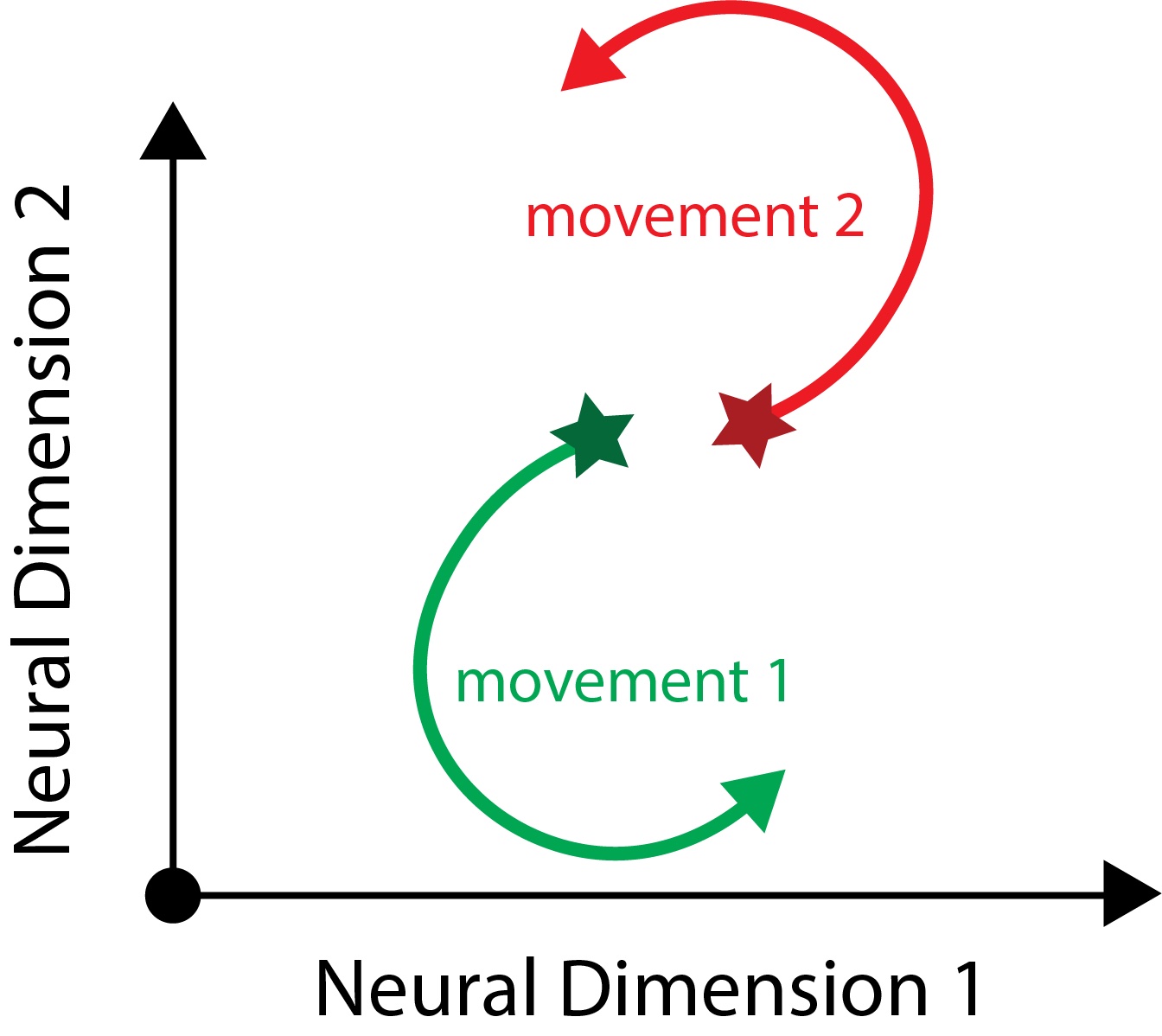
Figure 4. (a, left) Trajectories recorded during reaching tasks show clear rotational structure when visualised in two dimensions. Different rotations correspond to differ- ent reaching movements, coloured red and green here. Figure is from Churchland et. al. 20126. (b, right) My mockup of the same result.
Another interesting example—and relevant for our interest in anxious thought loops—are the neural trajectories (from fMRI) of responses to both “threatening” and “safe” stimuli. Remarkably, in each case, the trajectories travel into completely different regions of state space. In this case, there is clearly a “safe landscape” and a “threat landscape,” and the full neural landscape can be thought of as the combination of these two.
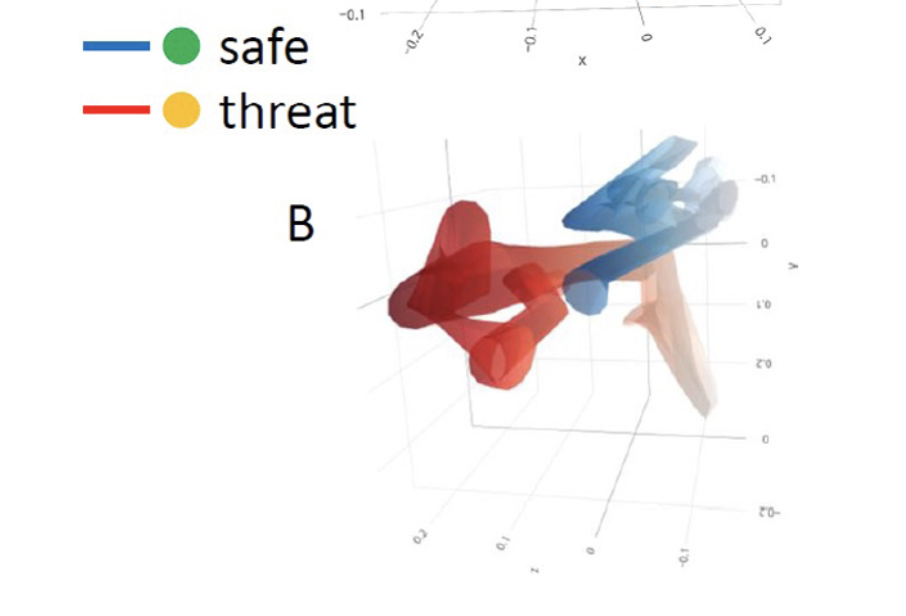
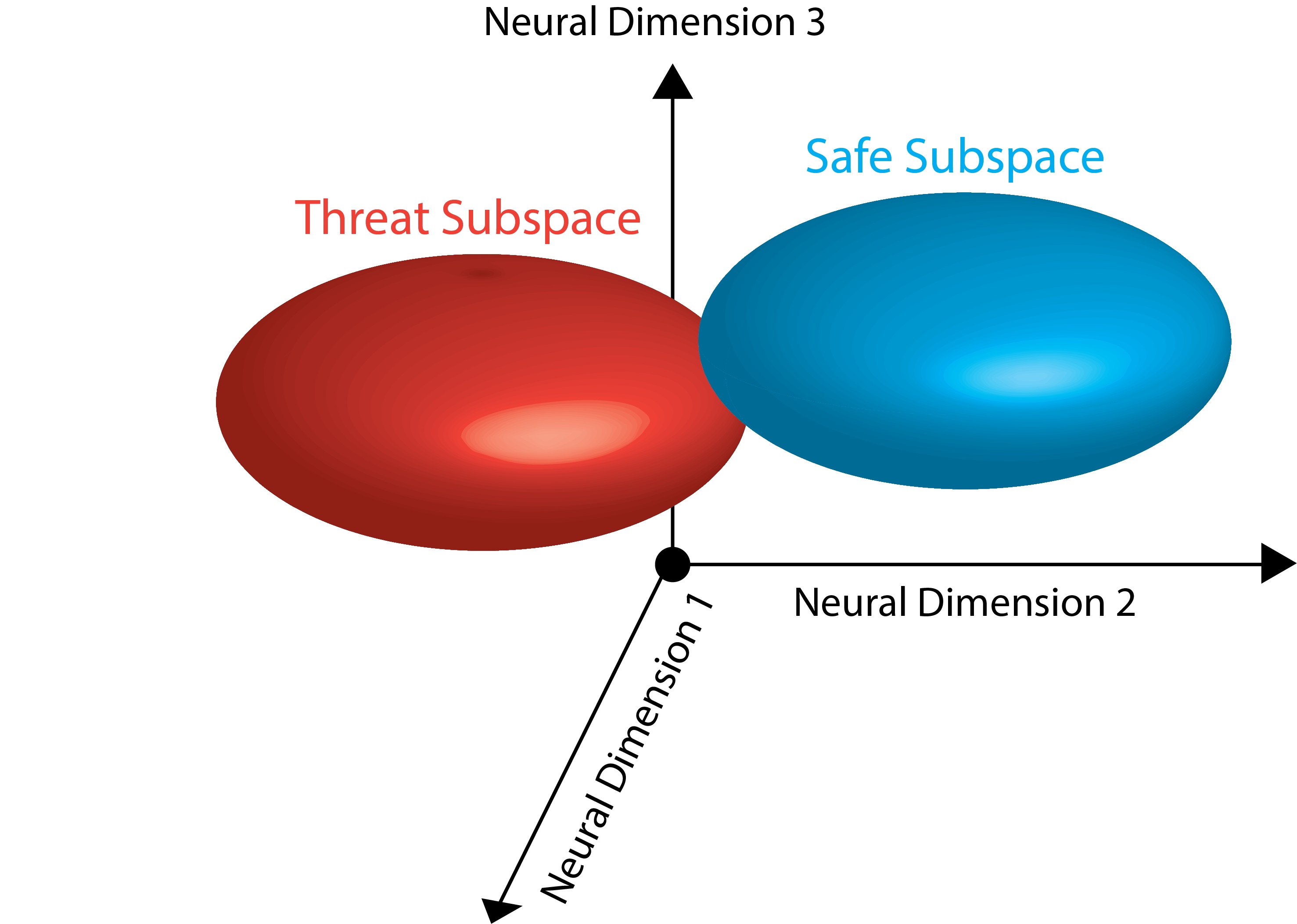
Figure 5. (a, left) Temporal trajectories from fMRI data in a study designed to elicit both threatening and safe experiences. Figure is from Pessoa 20197 using data from McMenamin et. al. 20148. (b, right) My mockup of the same result.
It’s natural to investigate trajectories in state space in a situation like this reaching example, or the threat example, where there is a clear task or quantity that might be represented in the state space. But what about when there is no task being performed? Are there general ways to characterise what’s happening in the neural state space?
One approach is to borrow a concept often used to think about systems that change over time: stability. In a stable system, the trajectory the system is travelling on is robust to perturbations or changes. Examples of stable systems are a pendulum with friction, always ending up at the bottom no matter where it is dropped from, or a person walking, able to recover their stride even if they momentarily trip. In an unstable system, however, even small changes or perturbations can massively change what happens in the system. The weather, for instance, is often thought to be an unstable system—and it has been suggested that even the flap of a butterfly’s wings in one location could cause a distant tornado9.
To bring this intuition into our neural state space, let’s pretend for a minute that the trajectories in neural state space evolve on a landscape that obeys the laws of gravity (I know, bear with me). If this landscape consists of two valleys, intuition tells us that if our trajectory starts anywhere on the edge of one of the valleys, it will end up at the bottom. To motivate this, let’s say ending up at the bottom of one valley corresponds to thinking about the concept “dog” and the other valley corresponds to the concept “cat.” When prompted with differing cues—say, a picture of a dog and a picture of a cat—you can easily tell them apart, even if the photos are a bit faded and grainy. Start your neural trajectory anywhere in the valley, and you end up at the bottom, thus “retrieving” the concept from your memory10.
The stability of this system corresponds to how deep the valleys are. If the valleys are deep, a distraction, or small change in the trajectory—say, you just remembered how hungry you are—will not cause you to identify the wrong animal (this involves more stability). But if the valleys are shallow, a small change in the trajectory could suddenly knock you into the other valley—and then who knows what could happen! (This would be more unstable, or less stability.)
One approach is to borrow a concept often used to think about systems that change over time: stability. In a stable system, the trajectory the system is travelling on is robust to perturbations or changes. Examples of stable systems are a pendulum with friction, always ending up at the bottom no matter where it is dropped from, or a person walking, able to recover their stride even if they momentarily trip. In an unstable system, however, even small changes or perturbations can massively change what happens in the system. The weather, for instance, is often thought to be an unstable system—and it has been suggested that even the flap of a butterfly’s wings in one location could cause a distant tornado9.
To bring this intuition into our neural state space, let’s pretend for a minute that the trajectories in neural state space evolve on a landscape that obeys the laws of gravity (I know, bear with me). If this landscape consists of two valleys, intuition tells us that if our trajectory starts anywhere on the edge of one of the valleys, it will end up at the bottom. To motivate this, let’s say ending up at the bottom of one valley corresponds to thinking about the concept “dog” and the other valley corresponds to the concept “cat.” When prompted with differing cues—say, a picture of a dog and a picture of a cat—you can easily tell them apart, even if the photos are a bit faded and grainy. Start your neural trajectory anywhere in the valley, and you end up at the bottom, thus “retrieving” the concept from your memory10.
The stability of this system corresponds to how deep the valleys are. If the valleys are deep, a distraction, or small change in the trajectory—say, you just remembered how hungry you are—will not cause you to identify the wrong animal (this involves more stability). But if the valleys are shallow, a small change in the trajectory could suddenly knock you into the other valley—and then who knows what could happen! (This would be more unstable, or less stability.)
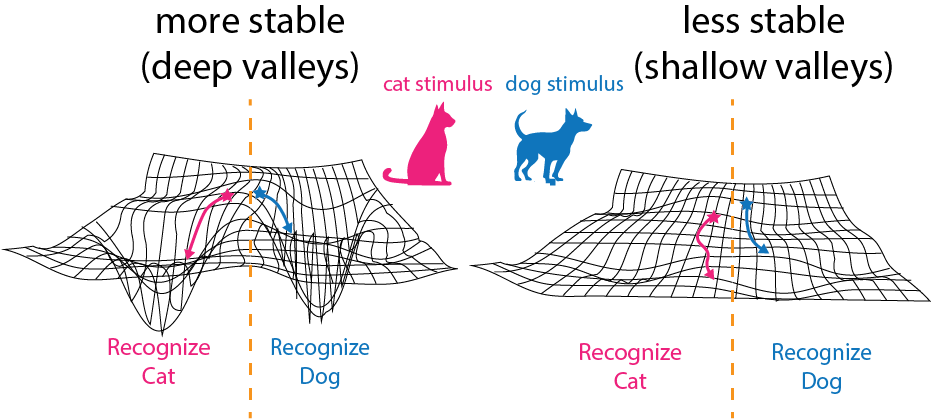
Figure 6. Examples of more stable and less stable landscapes. In a more stable landscape, deep valleys mean that the trajectories of the system are very reliable. In a less stable landscape, shallow valleys mean that the trajectories are more fragile and can be easily perturbed off course. This may affect computations performed by the brain, such as object recognition.
Researchers have suggested that the stability pres- ent in your brain could be directly related to your conscious state11-13. For instance, psychedelics are thought to destabilise the brain, and hallucina- tions might be your neural trajectory getting “bumped” from one valley to another14. On the other hand, conditions such as anxiety, depres- sion, OCD, and addiction, characterised by fixed negative thinking, are thought to be more stable relative to neurotypical brains15-17. These brains get stuck at the bottom of valleys corresponding to certain thoughts.
Which brings us back to waiting for a text to arrive. All those stubborn thoughts you’re having about why it hasn’t yet reached your phone? Maybe your neural trajectory is stuck at the bottom of a very, very deep valley. And no distraction—or change in your trajectory—can help you get out of it. In fact, when you try to distract yourself, you might even feel yourself mentally slipping back down the slope of the valley towards those pesky thoughts
This is all a hypothesis, of course. But the hope is that by measuring stability in neural data, and by identifying changes that have occurred due to specific treatments (for instance, meditation may help anxiety by enabling you to drag your neural trajectory out of a deep valley and towards something else—like your breath), we will be able to identify when people need help and what sorts of things might actually benefit them.
For now, though, instead of getting lost in thought about your absent text, see if you can bring some awareness to the feeling of being stuck in this valley of thought, and realise that there is no way to think yourself out of that cavernous depth. Just bring your attention to your breath, and slowly—but surely—climb your way out.
This is all a hypothesis, of course. But the hope is that by measuring stability in neural data, and by identifying changes that have occurred due to specific treatments (for instance, meditation may help anxiety by enabling you to drag your neural trajectory out of a deep valley and towards something else—like your breath), we will be able to identify when people need help and what sorts of things might actually benefit them.
For now, though, instead of getting lost in thought about your absent text, see if you can bring some awareness to the feeling of being stuck in this valley of thought, and realise that there is no way to think yourself out of that cavernous depth. Just bring your attention to your breath, and slowly—but surely—climb your way out.
REFERENCES
1. Lydon-Staley, D. M. et al. Repetitive negative thinking in daily life and functional connectivity among default mode, fronto-parietal, and salience networks. Transl. Psychiatry 9, 234 (2019).
2. Ruscio, A. M., Seitchik, A. E., Gentes, E. L., Jones, J. D. & Hallion, L. S. Perseverative thought: a robust predictor of response to emotional challenge in generalized anxiety disorder and major depressive disor- der. Behav. Res. Ther. 49, 867–874 (2011).
3. Ehring, T. & Watkins, E. R. Repetitive Negative Thinking as a Transdiagnostic Process. Int. J. Cogn. Ther. 1, 192–205 (2008).
4. Cunningham, J. P. & Yu, B. M. Dimensionality reduction for large-scale neural recordings. Nat. Neurosci. 17, 1500–1509 (2014).
5. Chaudhuri, R., Gerçek, B., Pandey, B., Peyrache, A. & Fiete, I. The intrinsic attractor manifold and population dynamics of a canonical cognitive circuit across waking and sleep. Nat. Neurosci. 22, 1512– 1520 (2019).
6. Churchland, M. M. et al. Neural population dynamics during reaching. Nature 487, 51–56 (2012).
7. Pessoa, L. Neural dynamics of emotion and cognition: From trajectories to underlying neural geometry. Neural Netw. 120, 158–166 (2019)
8. McMenamin, B. W., Langeslag, S. J. E., Sirbu, M., Padmala, S. & Pessoa, L. Network organization unfolds over time during periods of anxious anticipation. J. Neurosci. 34, 11261–11273 (2014)
9. Lorenz, E. N. Deterministic Nonperiodic Flow. J. Atmos. Sci. 20, 130–141 (1963).
10. Hopfield, J. J. Neural networks and physical systems with emergent collective computational abilities. Proc. Natl. Acad. Sci. U. S. A. 79, 2554–2558 (1982).
11. Carhart-Harris, R. L. et al. The entropic brain: a theory of conscious states informed by neuroimaging research with psychedelic drugs. Front. Hum. Neurosci. 8, 20 (2014).
12. Zimmern, V. Why Brain Criticality Is Clinically Relevant: A Scoping Review. Front. Neural Circuits 14, 54 (2020).
13. Toker, D. et al. Consciousness is supported by near-critical slow cortical electrodynamics. Proc. Natl. Acad. Sci. U. S. A. 119, (2022).
14. Carhart-Harris, R. L. & Friston, K. J. REBUS and the Anarchic Brain: Toward a Unified Model of the Brain Action of Psychedelics. Pharmacol. Rev. 71, 316–344 (2019).
15. Holtzheimer, P. E. & Mayberg, H. S. Stuck in a rut: rethinking depression and its treatment. Trends Neurosci. 34, 1–9 (2011).
16. Zullino, D. F. & Khazaal, Y. The ‘rut metaphor’: a conceptualization of attractor-shaping properties of addictive drugs. Subst. Use Misuse 43, 469–479 (2008).
17. Carhart-Harris, R. L. et al. Canalization and plasticity in psychopathology. Neuropharmacology 226, 109398 (2023).
2. Ruscio, A. M., Seitchik, A. E., Gentes, E. L., Jones, J. D. & Hallion, L. S. Perseverative thought: a robust predictor of response to emotional challenge in generalized anxiety disorder and major depressive disor- der. Behav. Res. Ther. 49, 867–874 (2011).
3. Ehring, T. & Watkins, E. R. Repetitive Negative Thinking as a Transdiagnostic Process. Int. J. Cogn. Ther. 1, 192–205 (2008).
4. Cunningham, J. P. & Yu, B. M. Dimensionality reduction for large-scale neural recordings. Nat. Neurosci. 17, 1500–1509 (2014).
5. Chaudhuri, R., Gerçek, B., Pandey, B., Peyrache, A. & Fiete, I. The intrinsic attractor manifold and population dynamics of a canonical cognitive circuit across waking and sleep. Nat. Neurosci. 22, 1512– 1520 (2019).
6. Churchland, M. M. et al. Neural population dynamics during reaching. Nature 487, 51–56 (2012).
7. Pessoa, L. Neural dynamics of emotion and cognition: From trajectories to underlying neural geometry. Neural Netw. 120, 158–166 (2019)
8. McMenamin, B. W., Langeslag, S. J. E., Sirbu, M., Padmala, S. & Pessoa, L. Network organization unfolds over time during periods of anxious anticipation. J. Neurosci. 34, 11261–11273 (2014)
9. Lorenz, E. N. Deterministic Nonperiodic Flow. J. Atmos. Sci. 20, 130–141 (1963).
10. Hopfield, J. J. Neural networks and physical systems with emergent collective computational abilities. Proc. Natl. Acad. Sci. U. S. A. 79, 2554–2558 (1982).
11. Carhart-Harris, R. L. et al. The entropic brain: a theory of conscious states informed by neuroimaging research with psychedelic drugs. Front. Hum. Neurosci. 8, 20 (2014).
12. Zimmern, V. Why Brain Criticality Is Clinically Relevant: A Scoping Review. Front. Neural Circuits 14, 54 (2020).
13. Toker, D. et al. Consciousness is supported by near-critical slow cortical electrodynamics. Proc. Natl. Acad. Sci. U. S. A. 119, (2022).
14. Carhart-Harris, R. L. & Friston, K. J. REBUS and the Anarchic Brain: Toward a Unified Model of the Brain Action of Psychedelics. Pharmacol. Rev. 71, 316–344 (2019).
15. Holtzheimer, P. E. & Mayberg, H. S. Stuck in a rut: rethinking depression and its treatment. Trends Neurosci. 34, 1–9 (2011).
16. Zullino, D. F. & Khazaal, Y. The ‘rut metaphor’: a conceptualization of attractor-shaping properties of addictive drugs. Subst. Use Misuse 43, 469–479 (2008).
17. Carhart-Harris, R. L. et al. Canalization and plasticity in psychopathology. Neuropharmacology 226, 109398 (2023).